Prediction and design
EdaFold
Fragment assembly is a powerful method of protein structure prediction that builds protein models from a pool of candidate fragments taken from known structures. Stochastic sampling is subsequently used to refine the models. We have developed a new method for fragment-based protein structure prediction based on an Estimation of Distribution Algorithm called EdaFold. This algorithm learns from previously generated decoys and steers the search toward native-like regions. A comparison with Rosetta AbInitio protocol shows that EdaFold is able to generate models with lower energies and to enhance the percentage of near-native decoys on a benchmark of 20 proteins.
Estimation of distribution algorithms (EDAs) are evolutionary algorithms. In these population-based meta heuristics, solutions share some information about promising regions of the search space in order to converge toward the global optimum. While in classical evolutionary approaches newly sampled solutions are generated by explicit recombination of the population's best solutions, EDAs strategy is to regenerate a pool of solutions with an estimated biased distribution. The distribution is biased by observation of the search sub-space described by good solutions in the population. The method is schematically illustrated.
This work has been published in PLOS ONE (publication1, publication2).
Estimation of distribution algorithms (EDAs) are evolutionary algorithms. In these population-based meta heuristics, solutions share some information about promising regions of the search space in order to converge toward the global optimum. While in classical evolutionary approaches newly sampled solutions are generated by explicit recombination of the population's best solutions, EDAs strategy is to regenerate a pool of solutions with an estimated biased distribution. The distribution is biased by observation of the search sub-space described by good solutions in the population. The method is schematically illustrated.
This work has been published in PLOS ONE (publication1, publication2).
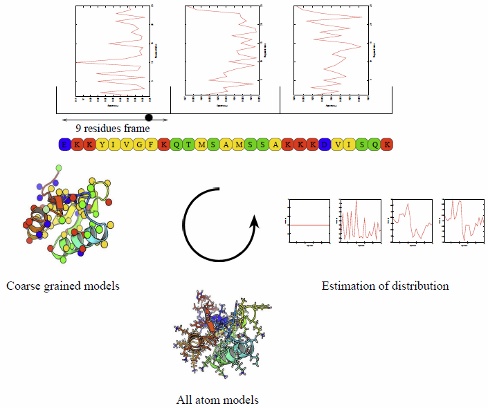